What Investors are Looking for in AI/ML
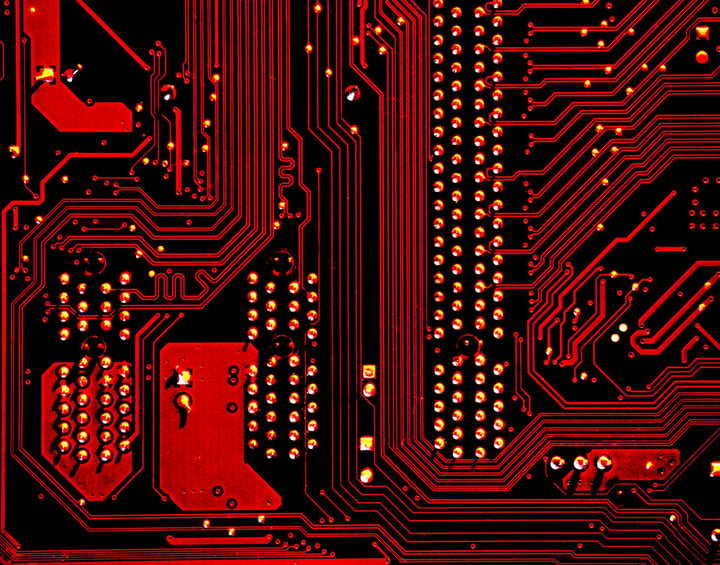
Advances in artificial intelligence (AI) have enabled machines to begin to outperform humans in areas like picking stocks, interpreting X-rays, and language translation. AI enables machines to perform human-like tasks with increasing degrees of autonomy by learning from experience and adjusting to new pieces of information.
Businesses are eager to use AI for new opportunities as the value of insights generated by AI is broadly understood. With the rise in interest around AI-powered applications, demand for tools and skills to analyze and utilize data generated by AI is growing rapidly. According to the International Data Corporation, total spend on AI across 19 industries was $37.5B in 2019 and is expected to reach nearly $100B in 2023.
We believe every industry can take advantage of AI opportunities across business functions, and we’ve invested behind this thesis. As we think about investing in AI/ML, we categorize our interest into four areas:
- Platform Tooling
- Enterprise Applications
- AI for Traditional Industries
- AI in Digital Health
Platform Tooling
In order to create AI applications, you first need a comprehensive suite of tooling. We categorize the AI/ML tooling pipeline in four main steps: setup, data prep, modeling & training, deployment.
Given that the quality of the data is crucial in creating an effective AI application, we’re excited by companies working in the data prep stage. Subcategories that interest us include data management, labeling, database, data generation, and processing. Unfortunately, each of these categories struggles with a variety of technical challenges given that new models are being deployed daily.
We also look for companies tackling problems in the deployment space, as we believe it’s the most undeveloped portion of the pipeline. Key tools include AI/ML lifecycle management to track and log dependencies since data scientists build multiple versions of a single model using different programming languages or libraries. Addressing communication issues is also key, as companies think about ways to increase collaboration between the data scientists and engineers deploying the models.
Enterprise Applications
As enterprise investors, we are looking for companies that take a “human-in-the-loop approach” with AI technologies, while ensuring both quality and scalability. The ability of AI to process huge amounts of data - much more than humans can - to recognize patterns, increase efficiency, and more makes them suitable for data-intensive applications.
For example, Text IQ uses machine learning to sift through sensitive data in corporate documents to find specific evidence or information. Without a product like Text IQ’s, tasks like these would have required hundreds, if not thousands, of hours of work.
Our investment in companies like Phenom People and Yalo present the use case for AI in the enterprise and leverage data streams to solve specific problems in verticals like human resources, customer success, and sales. We believe this trend will continue where AI will be uniquely tuned to solve problems in specific verticals.
According to our CXO Tech Survey, the second highest initiative among tech leaders across various industry clusters for 2020 was Enterprise AI.
We think about our investments in Enterprise AI based on the end-customer of the product and includes the following categories:
- Enterprise AI targeting back-office functions such as human resources, finance, and legal services.
- AI tools that are customer-facing such as chatbots, voice support, or personalization.
- Internal business tools aiming to improve productivity or efficiency.
AI for Traditional Industries
We also back entrepreneurs building companies that are applying novel AI techniques in traditional industries like manufacturing, industrials, and construction. High labor costs, along with increased competition, have forced these industries to take a more active approach in adopting AI across the value chain.
AI adoption also enables companies in these industries to improve their accuracy and efficiency. For example, Everest Labs leverages computer vision techniques to pick recyclables off a trash conveyor belt at a higher accuracy rate than traditional alternatives. AI tools can also be used to train workers in these industries, and even protect them. Invisible AI, for example, monitors assembly line workers using computer vision to watch for errors and identify problems that could result in an injury.
AI in Healthcare
AI adoption in the life sciences and healthcare industry has rapidly increased in the last decade. In the past, information was analog, but the digital transformation of the industry has generated an influx of data, including data generated by digitized hospital workflows, patient interactions, insurance claims, connected devices, and more.
Companies have taken advantage of the opportunities generated by the proliferation of data to address data issues in the healthcare system. For example, Deep Lens uses an integrated workflow and an analytics layer to find the best available clinical trials for a patient’s specific diagnosis.
We’re excited to meet the next wave of AI companies. If you’re an entrepreneur working on building the next great AI/ML company, reach out to us at marketing@sierraventures.com.
For more information about our Investment Thesis visit our Thesis Page.
Check out the AI/ML companies in our Portfolio.