Growing Applications of Synthetic Data
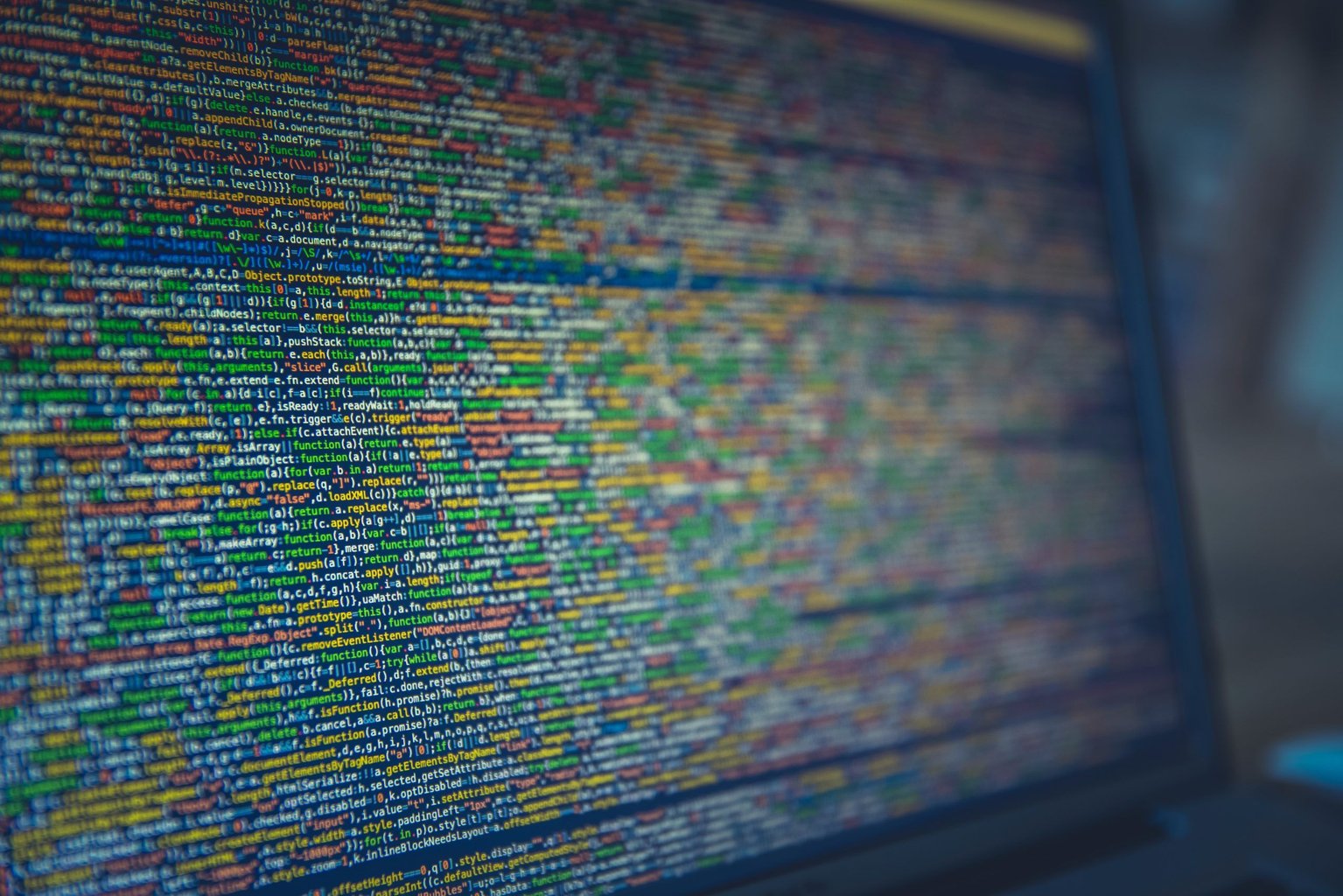
There has been growing interest in the emergence of synthetic data as a result of an abundance of low-cost computing and storage resources. Rather than being generated by real events, synthetic data is artificially-created datasets often comprising images or text that can be used for various applications in testing or across artificial intelligence.
Synthetic data is useful for:
- Testing new products and applications
- Training machine learning models
- Protecting privacy and confidentiality
Testing new products and applications
Developers can use synthetic data to provide more thorough testing of new products and product updates. Synthetic data is an attractive alternative to real data because developers don’t need to wait for the “real” data to be generated. This enables developers to decrease test time and push products to production faster. In addition, synthetic data allows unusual combinations of events to be produced, allowing developers to better test the reliability of their products and ensure a wider test scope.
Training machine learning models
Because large amounts of data are required to train machine learning models, synthetic data opens up new opportunities in AI. A model can be trained on a more comprehensive synthetically generated dataset before being applied to real data.
Synthetic data can also be automatically generated with little programming making it cheap and fast to produce. Synthetic data can also start with accurate labels, as compared to real data, which may require time and effort to accurately label the dataset. Lastly, synthetic data can be altered to adjust for bias and variance in the original, but sometimes limited, real dataset, enabling the model to be tuned for higher accuracy.
Protecting privacy and confidentiality
Real data often contains personal and confidential information that has restrictions around usage. With the onset of increased privacy regulations, companies can use synthetic data to reduce compliance concerns and protect sensitive data. Synthetic data can be modeled to remove personal information, so there is no tracing back to any individual. Therefore, companies can convert privacy-sensitive data into anonymous datasets while still maintaining the granular integrity of the data.
We’re excited about the potential of synthetic data and its applications to DevOps, machine learning, and security. If you’re working on a company in this space or have thoughts on where the space is headed, reach out to us at marketing@sierraventures.com.
Check out our take on the Opportunities we see in Machine Learning Operations.